2019年不可错过的45个AI开源工具,你想要的都在这里
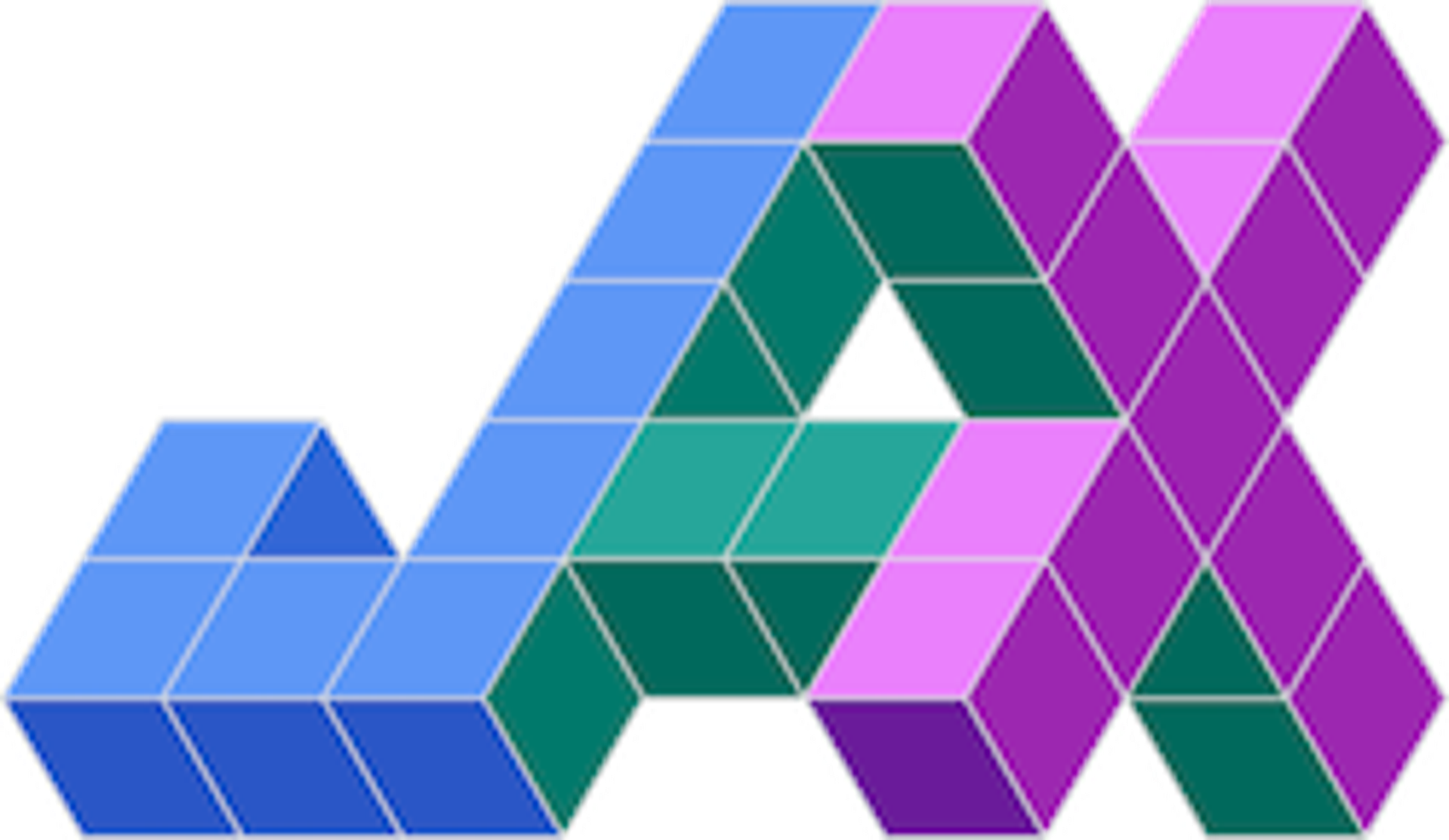
一个好工具,能提高开发效率,优化项目研发过程,无论是企业还是开发者个人都在寻求适合自己的开发工具。但是,选择正确的工具并不容易,有时这甚至是一项艰巨的任务。
2018年底,我们整理了去年备受关注的Python开源工具与项目Top100、
1、Jax【Stars:5.5k】
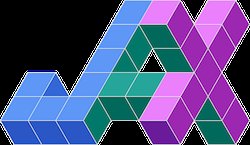
开源地址:https://github.com/google/jax
2、AdaNet【Stars:2.9k】
AdaNet是一款基于TensorFlow的轻量型框架。可以使用最少的专家干预来自动学习高质量模型,AdaNet提供的通用框架,不仅可以用于学习神经网络架构,还可以学习集成,从而获得更好的模型。
开源地址:https://github.com/tensorflow/adanet
TFX
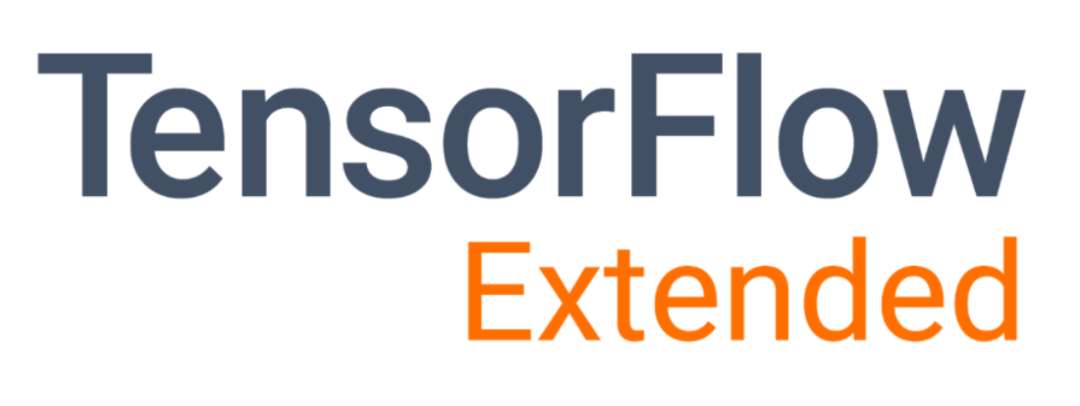
开源地址:https://github.com/tensorflow/tfx
TensorFlowFederated(TFF)框架可用于去中心化(decentralized)数据的机器学习及运算实验。它实现了联邦学习(FederatedLearning,FL)方法,将为开发者提供分布式机器学习,以便在没有数据离开设备的情况下,在多种设备上训练共享的ML模型。其中,它通过加密方式提供多一层的隐私保护,并且设备上模型训练的权重与用于连续学习的中心模型共享。
开源地址:https://github.com/tensorflow/federated
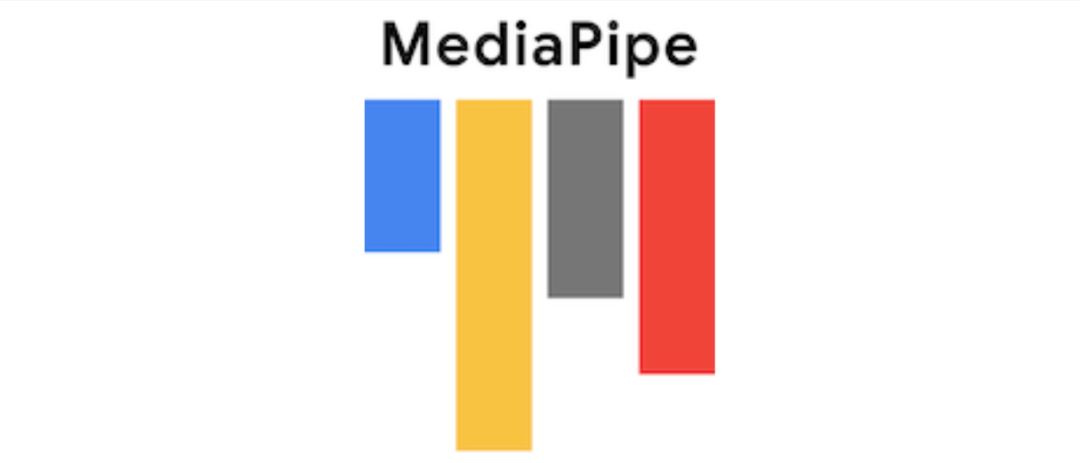
开源地址:https://github.com/google/mediapipe
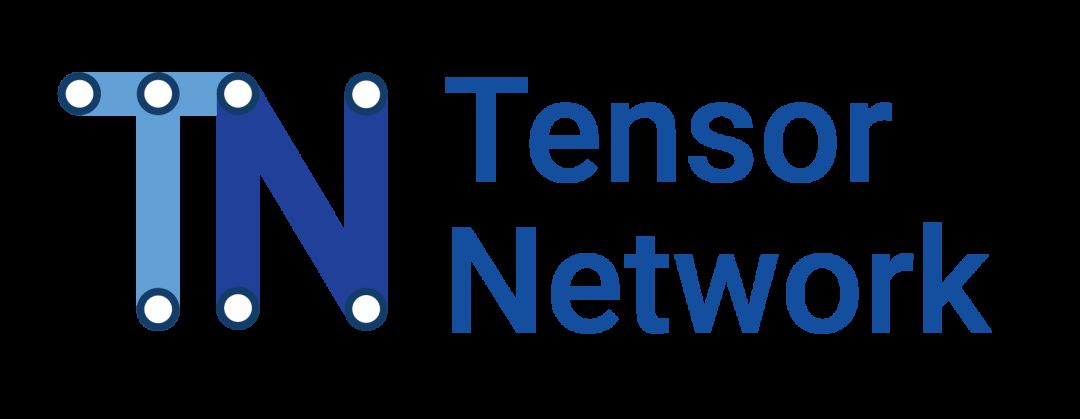
开源地址:https://github.com/google/tensornetwork
GPipe是一个分布式机器学习库,使用同步随机梯度下降和流水线并行技术进行训练,适用于任何由多个序列层组成的DNN。重要的是,GPipe让研究人员无需调整超参数,即可轻松部署更多加速器,从而训练更大的模型并扩展性能。
开源地址:https://github.com/tensorflow/lingvo/blob/master/lingvo/core/gpipe.py
MLIR通过定义一个通用的中间表示,将在TensorFlow和类似的ML框架中执行高性能机器学习模型所需的基础设施进行统一,包括高性能计算技术应用或强化学习这类搜索算法的集成。MLIR旨在降低开发新硬件的成本,并提高现有TensorFlow用户的可用性。
开源地址:https://github.com/tensorflow/mlir
1、OpenSpiel【Stars:1.7k】
OpenSpiel核心的OpenSpiel实现基于C++和Python绑定,这有助于在不同的深度学习框架中采用。该框架包含一系列游戏,允许DRLagent学会合作和竞争行为。同时,OpenSpiel还包括搜索、优化和单一agent等多种DRL算法组合。
开源地址:https://github.com/deepmind/open_spiel
开源地址:https://github.com/deepmind/spriteworld
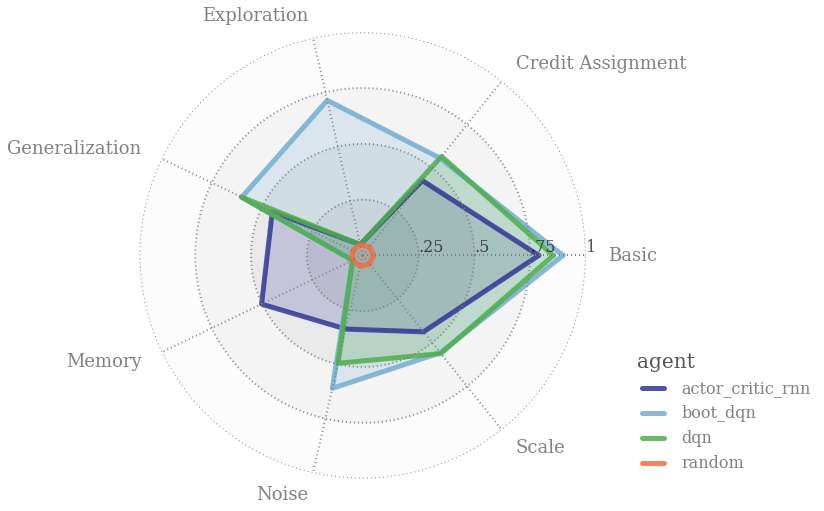
开源地址:https://github.com/deepmind/bsuite
全部开源:
https://ai.facebook.com/results/open-source/?content_types%5B0%5D=blog
1、LASER【Stars:2k】
增强版的LASER开源地址:https://github.com/facebookresearch/LASER
10月11日,Facebook发布了Detectron2,这是基于PyTorch框架,以maskrcnn-benchmark为起点对Detectron的彻底重写。通过全新的模块化设计,Detectron2灵活且可扩展,能够在单个或多个GPU服务器上提供更加快速的训练。
Detectron2已经包含了众多保质量实现的目标检测算法,包括:DensePose,panopticfeaturepyramidnetworks和MaskRCNN的各种变种。,其模块化特性也使其能够有效帮助研究人员探索最先进的算法设计。
开源地址:https://github.com/facebookresearch/detectron2
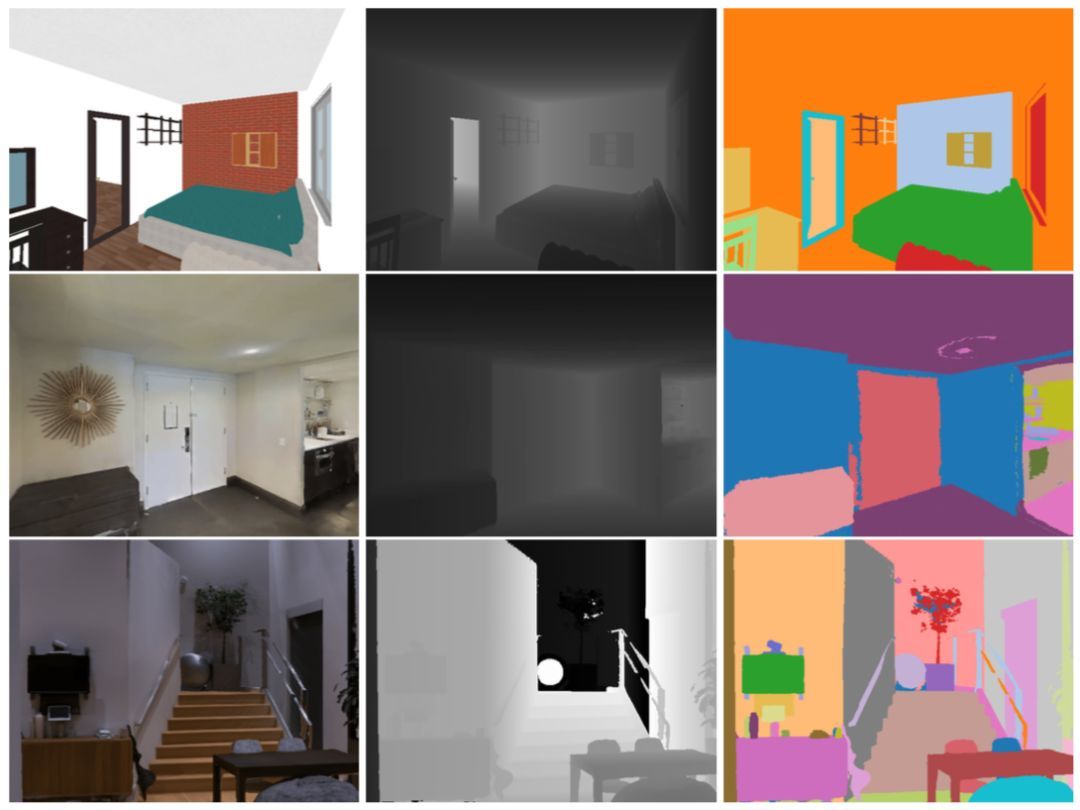
开源地址:https://github.com/facebookresearch/habitat-sim
PyText是一个基于PyTorch构建的NLP建模(基于深度学习)框架,核心功能可以支持文本分类、序列标注等神经网络模型。PyText可以简化工作流程,加速试验,同时还能促进大规模部署。
开源地址:https://github.com/facebookresearch/pytext
5、Nevergrad【Stars:2.1k】
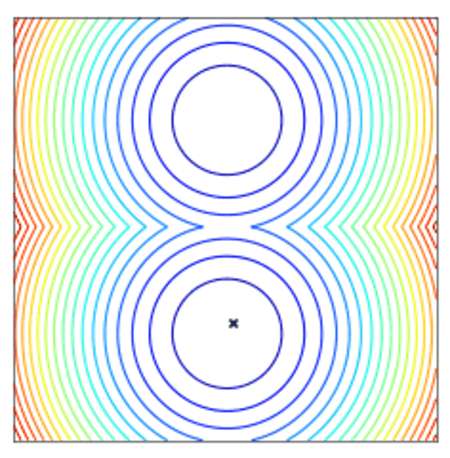
开源地址:https://github.com/facebookresearch/nevergrad
开源地址:https://github.com/pytorch/QNNPACK

开源地址:https://github.com/facebookresearch/PyTorch-BigGraph
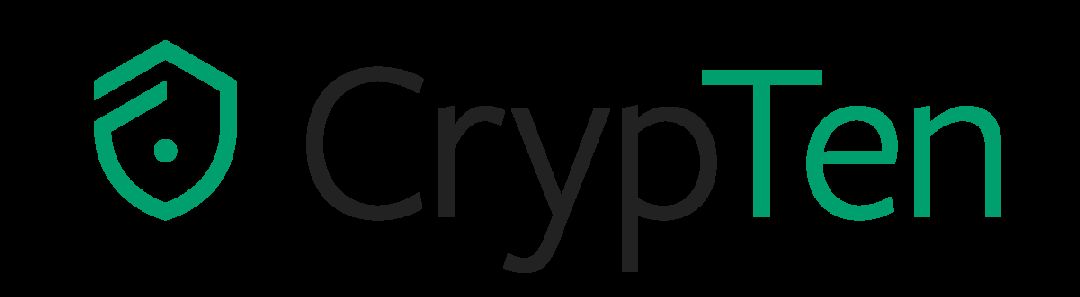
开源地址:https://github.com/facebookresearch/crypten
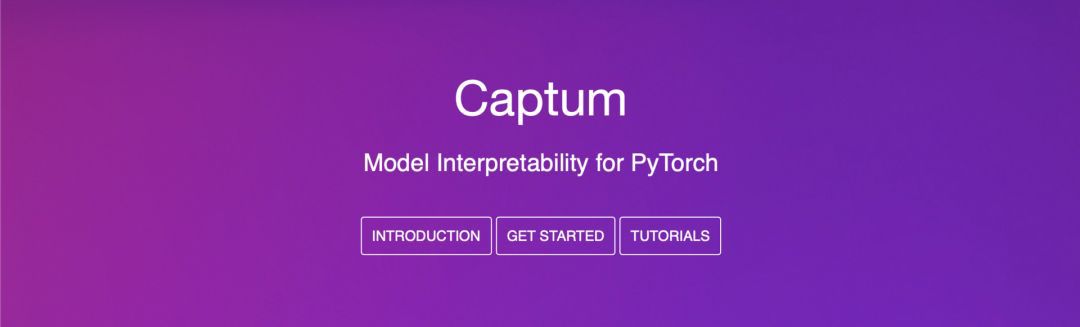
开源地址:https://www.captum.ai/
开源地址:https://github.com/facebookresearch/dlrm
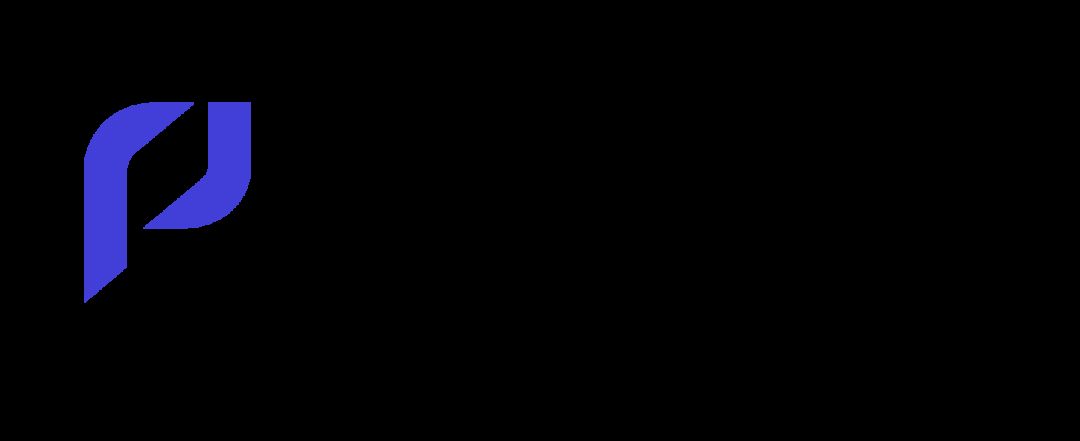
开源地址:https://github.com/facebookresearch/pythia
1、DGL【Stars:3.2k】
与传统基于张量(Tensor)的神经网络相比,图神经网络将图(Graph)作为输入,从图结构中学习潜在知识,该方法在近些年已被证明在许多场景可以取得很好的效果。然而,使用传统的深度学习框架(比如TensorFlow、Pytorch、MXNet)并不能方便地进行图神经网络的开发和训练,而DGL作为专门面向图神经网络的框架,可以很好地弥补这一缺陷。该框架在开源后于国内外引起了强烈的反响。
开源地址:http://dgl.ai
2、Neo-AI
开源地址:https://github.com/neo-ai
1、NNI(NeuralNetworkIntelligence)【Stars:3.5k】
NNI开源地址:https://github.com/microsoft/nni/blob/master/README_zh_CN.md
开源地址:https://github.com/microsoft/pai/blob/master/README.md
开源地址:https://github.com/interpretml/interpret
开源地址:https://github.com/msr-fiddle/pipedream
1、Ludwig【Stars:5.9k】
开源地址:https://github.com/uber/ludwig
1、OpenVINO【Stars:873】
开源地址:https://github.com/opencv/dldt
1、TensorFlow.Net【Stars:807】
TensorFlow.NET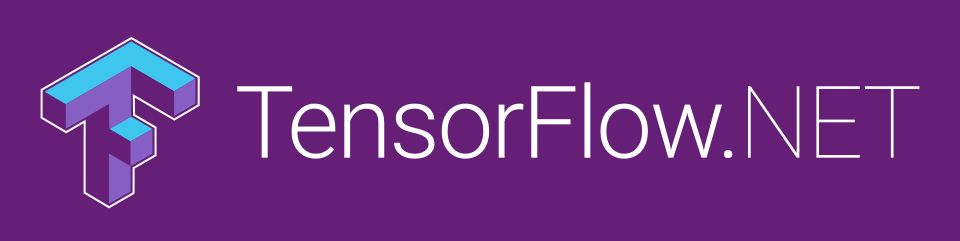
开源地址:https://github.com/SciSharp/TensorFlow.NET
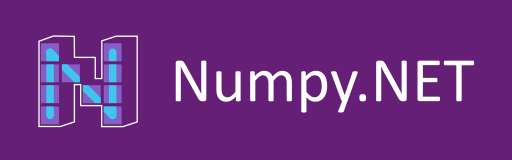
开源地址:https://github.com/SciSharp/Numpy.NET
1、Euler【Stars:1.8k】
Euler开源地址:https://github.com/alibaba/euler/wiki
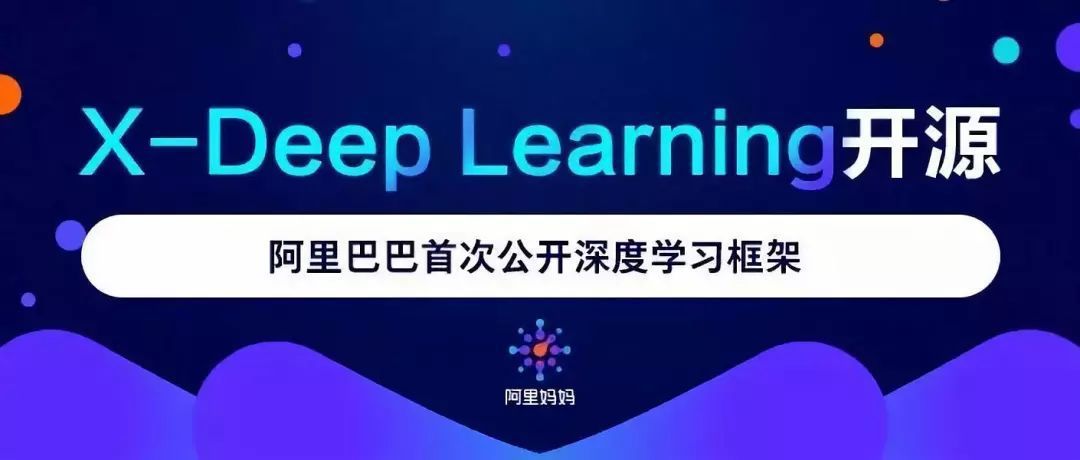
开源地址:https://github.com/alibaba/x-deeplearning
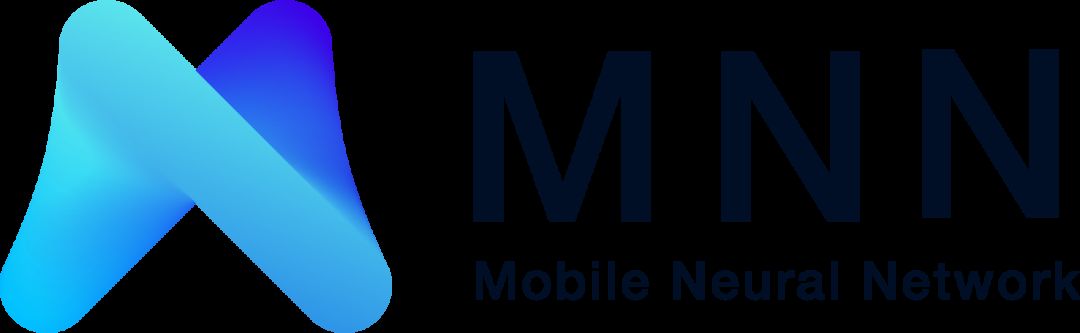
开源地址:https://github.com/alibaba/MNN/blob/master/README_CN.md
1、Angel【Stars:5.4k】
腾讯Angel3.0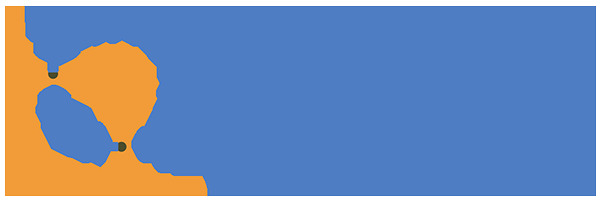
开源地址:https://github.com/Angel-ML/angel
1、BytePS【Stars:1.9k】
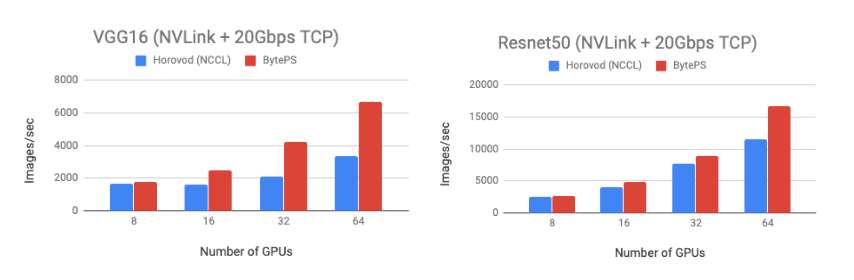
开源地址:https://github.com/bytedance/byteps
1、MMLabMMDetection【Stars:7k】
mmdetection开源地址:https://github.com/open-mmlab/mmdetection
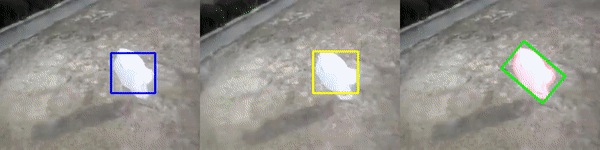
开源地址:https://github.com/STVIR/pysot
1、SMRC【Stars:547】
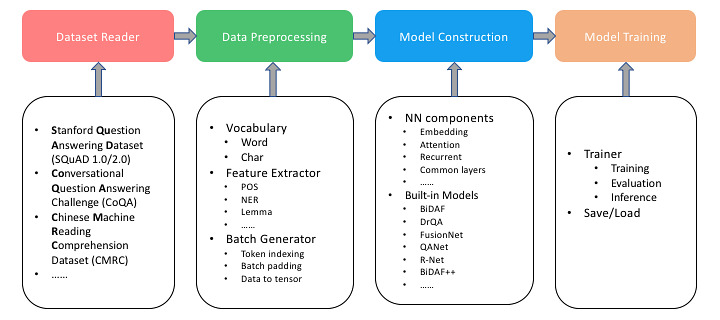
开源地址:https://github.com/sogou/SMRCToolkit
1、ElasticDL【Stars:355】
ElasticDL开源地址:https://github.com/sql-machine-learning/elasticdl/
1、FATE【Framework】
微众银行AI团队开源的联邦学习框架。联盟学习开源项目FATE(FederatedAITechnologyEnabler),提供了一种基于数据隐私保护的安全计算框架,为机器学习、深度学习、迁移学习算法提供强有力的安全计算支持。安全底层支持同态加密、秘密共享、哈希散列等多种多方安全计算机制,算法层支持多方安全计算模式下的逻辑回归、Boosting、联邦迁移学习等。
该框架可以帮助学术研究人员快速开发算法原型;为工业界人员快速开发应用提供一种简洁有效的解决方案,支持在多场景下的开拓和应用;借助其灵活的架构,用户可以轻松地将计算工作部署到多种平台(CPU、GPU)和设备(桌面设备、服务器集群、移动设备等)。
开源地址:
https://www.fedai.org.cn/cn/
https://github.com/FederatedAI/FATE
2、DeepCTR【Stars:2.2k】
开源地址:https://github.com/shenweichen/DeepCTR
开源地址:https://github.com/NUAA-AL/ALiPy
开源地址:https://github.com/CorentinJ/Real-Time-Voice-Cloning
5、WeightsandBiases【Stars:1.3k】

一个在机器学习实验过程中用于可视化和跟踪的工具。它与框架无关,比TensorBoard更轻。每次运行一个装有wandb的脚本时,都会保存超参数和输出度量。在训练过程中可视化模型,还能轻松对比模型的版本,还会自动跟踪你的代码、系统指标和配置参数的状态。
开源地址:https://github.com/wandb/client